Machine learning algorithm shows promise in early diagnosis of PAH
A recently developed machine-learning algorithm has shown potential in identifying patients at risk for pulmonary arterial hypertension (PAH) earlier in their symptom journey, according to a study. The algorithm aims to improve the timely diagnosis of PAH, which is associated with better patient outcomes and reduced healthcare costs.
By employing random forest models, the algorithm successfully classified patients as either PAH or non-PAH at the time of diagnosis and 6 months prior. The study included 1339 patients in the PAH cohort and 4222 patients in the non-PAH (control) cohort. The machine-learning model exhibited strong performance in distinguishing PAH patients from non-PAH patients, with an area under the curve of the receiver operating characteristic of 0.84, a recall (sensitivity) of 0.73, and a precision of 0.50 at 6 months before diagnosis.
Key differentiating factors between the PAH and non-PAH cohorts included a longer duration between the onset of symptoms and the prediagnosis model date, higher utilization of healthcare resources such as diagnostic and prescription claims, circulatory claims, imaging procedures, and increased hospitalizations.
Reference
Hyde B, Paoli CJ, Panjabi S, et al. A claims-based, machine-learning algorithm to identify patients with pulmonary arterial hypertension. Pulm Circ. 2023;13(2):e12237. doi: 10.1002/pul2.12237. PMID: 37287599; PMCID: PMC10243208.
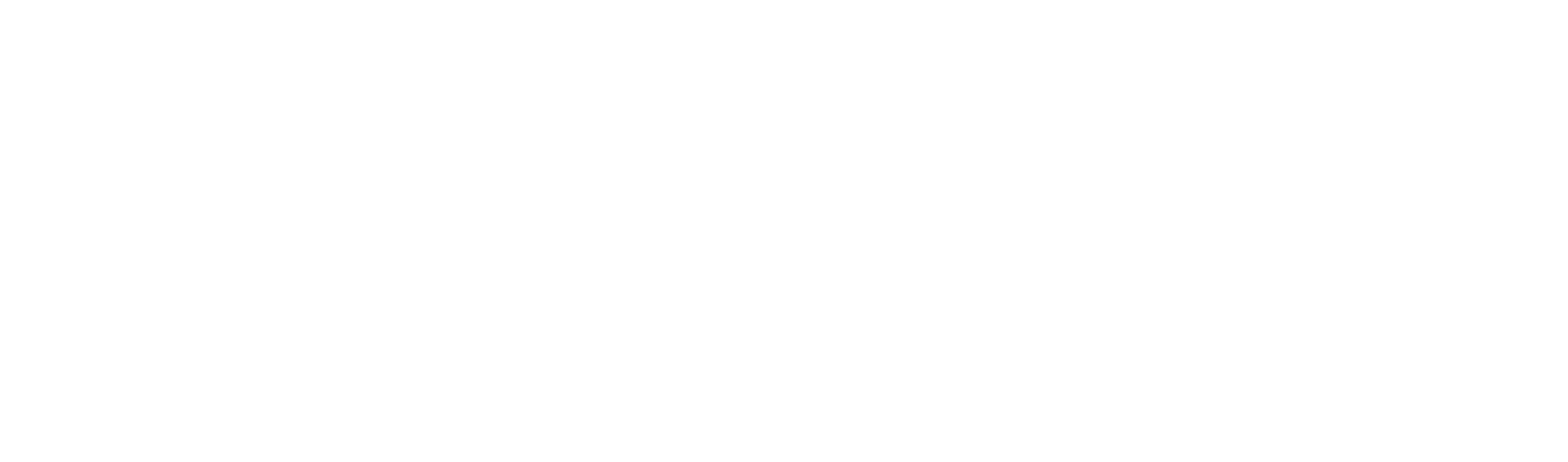